Remote Sensing 101
Introduction
Remote sensing is the science of gathering information about a location from a distance. For example, sensors on board satellites record the amount of emitted or reflected energy from the Earth’s surface, which can then provide information to scientists about the condition of the surface and provide insights to decision-makers.
Sensors
Sensors are the actual instruments that collect data about the objects they are observing by measuring the amount of energy returned to the instrument. Sensors are often designed for particular applications, such as monitoring the Earth’s temperature, land surface, or ocean floor, and thus are built to record specific information from different parts of the electromagnetic spectrum that are relevant to those applications.
Note
Read more about sensor types and what datasets are available through the Cartesian Workbench.
The Electromagnetic Spectrum
The electromagnetic (EM) spectrum describes the entire range of frequencies of EM radiation that exists. Different portions or classes of the EM spectrum can be broken out according to its frequency or wavelength, including gamma rays (short wavelength, high frequency), visible light (longer wavelength, lower frequency), and radio waves (longest wavelength, lowest frequency). Portions of the electromagnetic spectrum penetrate the atmosphere better than others, allowing earth observation sensors to effectively detect frequencies within the visible, infrared, and microwave spectrum.
Sensors are designed to record EM radiation within discrete ranges, or bands. For example, optical sensors often have bands representing red, green, and blue light. The exact range of wavelengths recorded for each band varies by sensor. For example, Sentinel 2’s red band covers a wavelength range of 650nm to 680nm, while Landsat 8’s red band records data in the range of 635.8nm to 673.35nm. Wavelengths and band lengths are typically expressed in nanometers or micrometers in remote sensing.
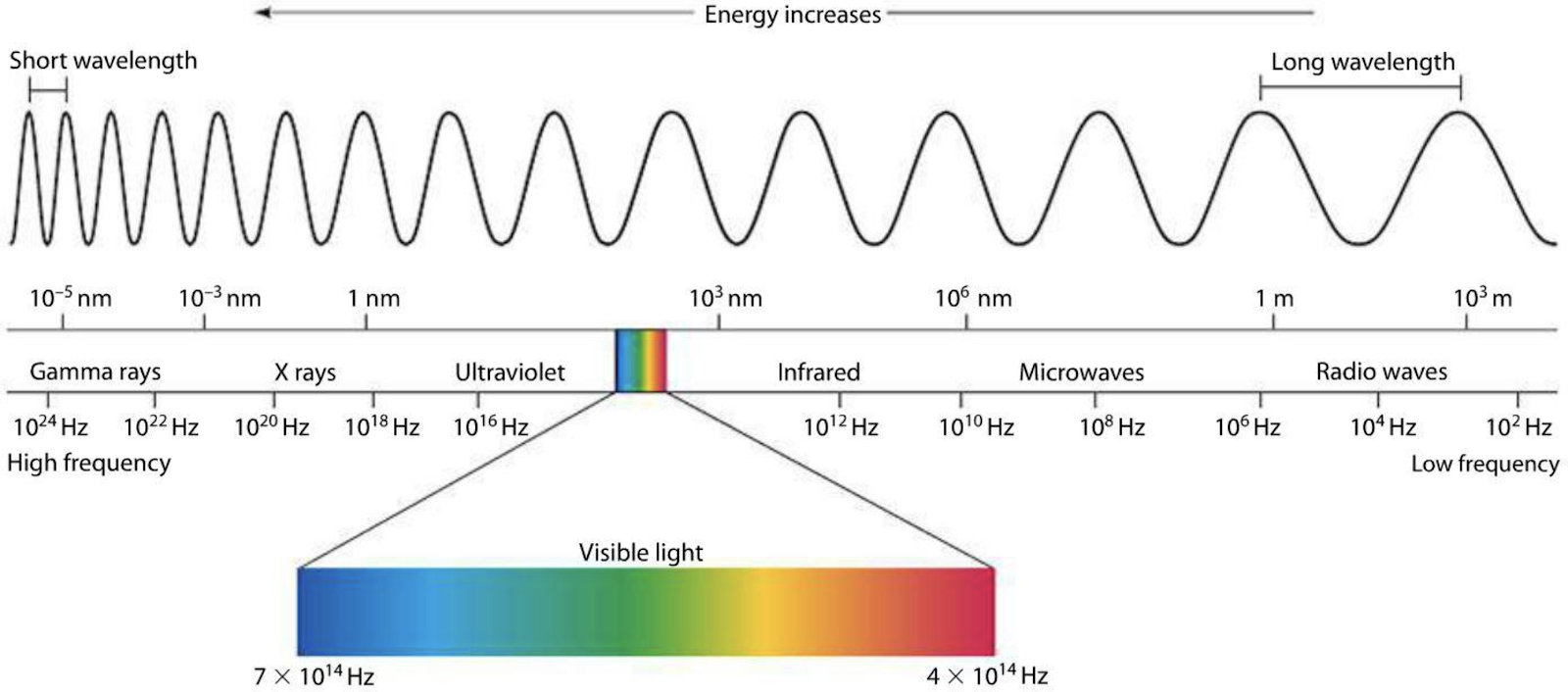
The Electromagnetic Spectrum: Remote sensing instruments are designed to capture specific ranges of the electromagnetic spectrum. miniphysics.com
Passive sensors
Passive systems, like Landsat and MODIS, measure the amount of electromagnetic energy being reflected from the Earth. We sometimes refer to these as optical sensors, as they detect reflected visible, near infrared, and short-wave infrared radiation. Various materials on the Earth’s surface reflect energy differently, allowing us to classify land cover and make other inferences about the planet using optical data.
Thermal remote sensors measure radiation being emitted from the earth’s surface or a target body, rather than reflected in the case of optical sensors described above. Thermal energy registers in the thermal infrared region of the electromagnetic spectrum and can be used to make temperature measurements of the land or sea surface. Applications include measuring the presence of irrigation leaks in agricultural areas, modeling regional energy balances between land surfaces and water bodies, and fire detection.
Active sensors
Active sensors (like radar or lidar) do not rely on energy from the Sun or Earth’s thermal properties. Instead, active remote sensing sensors transmit energy toward the Earth’s surface, which then interacts with the terrain and returns back to the sensor. Several active remote sensing platforms use radar to scan the Earth’s surface. Radar has a longer wavelength when compared to visual light, causing it to have atmosphere and cloud-penetrating properties. Thus, radar data can be especially useful for monitoring tropical areas that are covered in clouds much of the year.
Note
So far, work at Descartes Labs has used radar (e.g. Sentinel 1A), which transmits long wavelength microwaves (i.e. 0.75 - 25 cm), and LiDAR, which uses short wavelength laser light (i.e. 0.90 \(\mu\)m).
Platforms
Platforms are the objects that carry sensors. These can be stationary, moving linearly, or orbiting. Many of the datasets hosted by Descartes Labs collect imagery from sensors mounted on satellites in orbit. Others were collected on special missions by attaching sensors to airplanes. Satellite platforms can be in several types of orbits depending on their mission. Most Earth observation satellites are in Sun synchronous, low Earth orbit (Landsat, Sentinel). Sun synchronous orbits allow a satellite to move with the Sun, maintaining a constant illumination angle as it images the Earth.
For satellites that need to maintain a consistent view (weather satellites), a geostationary orbit keeps the sensor over the same location at all times. Geostationary orbits are often used to collect data over large areas of the Earth very frequently. Airborne platforms allow for greater flexibility with regards to how imagery is collected, and can include airplanes, drones, or balloons. Imagery can also be collected using ground-based platforms such as hand-held devices, tripods, towers, or cranes.
Resolutions
Remote sensing datasets have three main types of resolution associated with them: spatial, temporal, and spectral. The choice of which resolutions are the most important is dependent on the user’s application.
Computer vision (CV): A user is interested in counting the number of swimming pools in southeastern Florida. This will require images that can resolve relatively small objects to allow the CV model to identify the pools (spatial resolution), but likely will not require information from bands beyond the visible range (spectral resolution), or require multiple images of the study area (temporal resolution). Overall, the required spatial resolution will be high, while the spectral and temporal resolution requirements will be low. An ideal sensor here may be Airbus SPOT or Pléiades imagery.
Vegetation Monitoring: A land manager is interested in monitoring vegetation growth over the course of the year. In order to separate healthy vegetation from areas with little to no growth, the sensor needs to measure bands covering various parts of the EM spectrum that are sensitive to vegetative growth, from the red to near/mid infrared regions. As the user is interested in getting regular updates throughout the year, the revisit rate (temporal resolution) should be relatively high. However, since the user is interested in broader spatial trends, very high spatial resolution is less important. In this case, Sentinel-2 would work well.
Wildfire Detection: A user is interested in detecting fires across North America. In this case, there is a need to get very frequent updates (temporal resolution). The spectral range needs to include bands that are sensitive to high temperatures (midwave-IR, thermal-IR, and shortwave-IR). Given the large area of interest, the user is satisfied using data without very high spatial detail. For this application the GOES sensor, which images the western hemisphere every 15 minutes, may be a good fit.
Generally speaking, users have to compromise on some element of resolution. Technical limitations prevent a single sensor from capturing data at high resolution in all three areas. Increasingly, with access and the ability to take advantage of more diverse and larger datasets, a user may achieve high resolution across all three areas by combining data from different sensors.
Spatial resolution refers to the size of the ground element represented by a single pixel. Generally, this is expressed as the size of a single pixel along one side, e.g. the spatial resolution of the Sentinel-2 red band is 10 meters. Most imagery in the Landsat archive has a spatial resolution of 30 meters. At this resolution, one pixel is the size of a baseball infield, however, to reliably detect an object in an image it’s often recommended that the object should occupy at least 3-9 pixels, depending on the sensor. Spatial resolution has major implications on the size of the data stored on disk, legality of use, ease of access and cost, and most importantly, what you can distinctly identify from the imagery. If a user needs to count cars, Landsat will not meet their spatial resolution needs, and they may need to save room in their project’s budget for high resolution data.
Spectral resolution refers to the sensitivity of the sensor to resolve fine ranges in the EM spectrum. A sensor with high spectral resolution will contain information about very narrow spectral ranges (e.g. each band may cover a 10nm range), while a sensor with a coarse spectral resolution could contain a bands with information about a wide spectral region (e.g. the entire visual range, about 400nm). Differences in spectral range and resolution between satellites can have a meaningful impact. It is particularly important for applications where the feature of interest has a narrow absorption feature, such a mineral mapping, meaning that signal of the feature is only distinct in a narrow range of EM radiation.
Temporal resolution refers to the frequency of image updates for any given location on the Earth. This is also called the satellite “revisit rate”. Satellites that are collecting in sun-synchronous orbit will have a regular revisit rate over a given location on Earth (e.g. Sentinel-2 will revisit a location either every 5-10 days depending on the location), while satellites that must be tasked or aerial datasets will have more irregular collection patterns. Temporal resolution is important for monitoring applications, as well as for applications where the temporal pattern of the signal is informative. For example, high temporal resolution is often important for agricultural applications, where the satellite can be used to track the growth phases of a crop over the growing season.
Image Correction
For optical imagery, the most scientifically useful quantity is \(\rho\), the percentage of incident radiation (\(I\)) that is reflected from the surface and detected by the sensor (radiant flux \(R\)). Conceptually, this is the fraction of the Sun’s light that is reflected by the Earth’s surface.
\(\rho\prime = R / I\)
For a given spectral range, I can be estimated directly from a reference Solar irradiance spectra. R is generally calculated directly from the raw sensor data (e.g. digital number), using the sensor specific conversion formula. To correct for the illumination angle of the sun (\(\theta\)), \(\rho\prime\) can be adjusted based on a reflectance model. The simplest model, Lambertian, removes illumination effects using a cosine correction:
\(\rho = \rho\prime / cos(\theta)\)
The imagery is now considered corrected to ‘top of atmosphere’ (TOA), meaning that the data are adjusted to model the reflectance measurement at the top of the atmosphere. At this point the imagery needs to be corrected for the effects of terrain (orthorectification) and distortion resulting from the signal passing through the atmosphere (atmospheric correction). Often the illumination correction is done at the same time as the terrain correction, as the illumination angle of each pixel may be different in rugged areas.
Note
All imagery offered through the Descartes Labs data refinery has undergone some level of data correction described in this section, though the processing levels vary by sensor and band. All products have been georeferenced, optical imagery has been converted from raw digital numbers to top of atmosphere reflectance.
Orthorectification
Orthorectification is the process of removing topographic, illumination, and perspective effects from an image. When an image is collected, terrain (e.g. mountains and valleys) can cause some areas to receive different amounts of light, or to appear offset from their actual position. Orthorectification corrects these irregularities, using a digital elevation model of the area to normalize illumination across the scene and to remove distortions due to the terrain.
Atmospheric Correction
The atmosphere has an inherent influence on almost all remote sensing data. Atmospheric conditions can change the amount of electromagnetic radiation that reaches the Earth’s surface, and distort or diminish that radiation as it travels back through the atmosphere to a sensor. Atmospheric particles, the angle of the sun at the time the sensor was triggered, and the weather on a given day will all greatly impact the quality of an image. When a project requires utilizing imagery acquired under different times and conditions, it is essential to remove the effects of the atmosphere. When the effects of the atmosphere have been removed, the imagery has been modeled to surface reflectance, or the reflectance at which the sensor would record if it were just above the surface of the Earth.